Collaborative Innovations
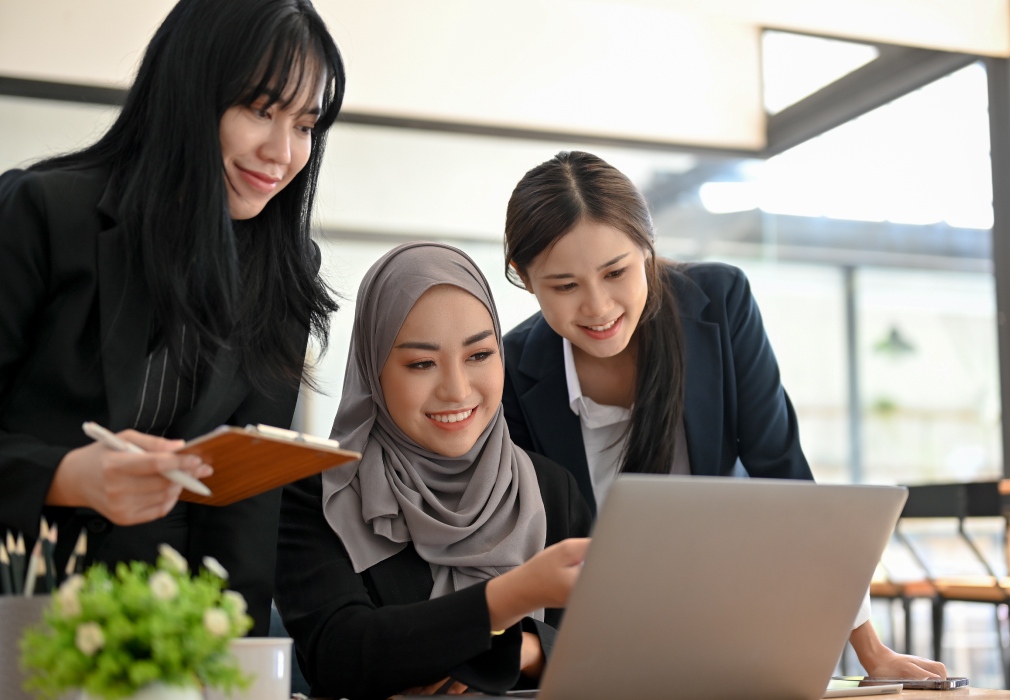
- Reducing Wind Noise for Better Two-Way Crisis Communications
- “Better Eyes” for Machines that See Micro – Cracks in Solar Wafers
- A 3-Musketeer Start-up Which Now Designs ICS for the Global Market
- The Australian Inventor Who Brought His AI-Driven Stethoscope Project to Penang
A novel approach for prioritization of product quality improvement in a complex manufacturing environment.
Product quality is critical for business organisations due to a highly competitive, customer driven market. Robust products and reliable services play a key role in ensuring long-term customer loyalty. The aim of our project is to design an information system that will assist in prioritizing product quality improvement based on available data.
On the onset of our project, we began looking at our study from a high-level systemic view. We then took a data modelling approach to narrow down to potential focus areas for quality improvement. Further analysis was carried out to prioritise critical factors affecting specific product quality issues and subsequent actions were taken on the selected factors. Results were later monitored and impacts validated.
The value of research and innovation lies in solving real-life problems. I witnessed first hand the positive effects of embedding robotics as part of a healthcare solution and it inspired me to further my research and develop my own therapeutic robot for the Malaysian market”
Dr Yeong Che Fai, CREST funded researcher
The project was divided into four major phases. For phase one, we conducted a study on the product life cycle so as to define the entire scope of our study. In phase two, statistical or AI techniques were used to perform data analyses and in phase three, a prototype was created to manage and prioritize product quality improvement. Finally, in phase four, the impacts of the developed information system were assessed, and results were disseminated.
The achievements of this project include a Decision Support System to prioritize the critical parameters at the test stations to allow for early intervention using historical product testing data. A machine learning approach was then implemented, using historical data to support the decision-making process in parameter prioritisation based on data consistency. The prioritized parameters enable users to make intelligent decisions to identify failures to that can be solved at an early stage.